22/10/2024
CTI
Targeting AI-based user interfaces
Cybersecurity Insights
Data are known to be the black gold of the 21st century and arouse envy among attackers. The interfaces you consult each day undergo multiple assaults and their manipulation endangers lots of companies as well as their clients. The helpfulness of the little virtual assistant provided by your bank could well be used against you. Discover the different methods used to achieve this in this Insight written by the CWATCH team.
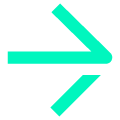
How are organisations affected?
Data poisoning refers to the intentional corruption or manipulation of training data used to develop machine learning models including those powering chatbots. But their implementation relies on large sets of data accessible by third parties. In this way, threat actors can, for example, use prompt injection to generate offensive content or even disclose confidential information which can have crippling consequences.
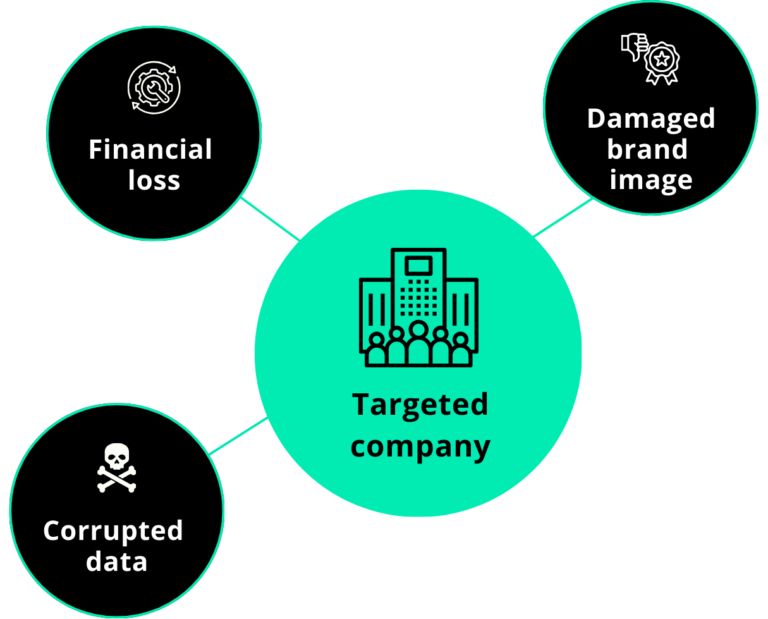
Concerns from all parties: privacy in peril
Conversely, chatbots can also be used to collect vast amount of data that can be manipulated to do profiling. They may be used for general inquiries but some of them are able to access private information to fulfil specific requests, especially when they are built to provide personalised assistance.
The relative absence of encryption, inadequate policies, vulnerabilities and not raising awareness among employees are the main weaknesses organisations should pay more attention to.
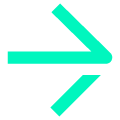
Compromising chatbots
- Alluring objectives
Chatbots are one of the most convenient benefits brought by AI to organisations from various horizons as they facilitate an organisation’s interactions with their customers. As a unique type of interface, they also enable threat actors to perform multiple malicious activities:
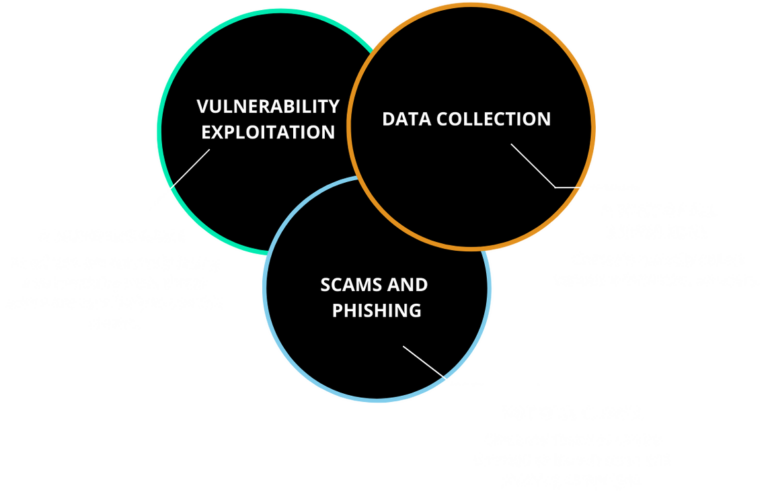
- Malware distribution
Taking advantage of chatbots’ weaknesses to carry out attacks. For instance, if a chat system permits users to share files, cybercriminals could take advantage of this capability to upload files containing malware.
Upon entering, they have the capability to breach the system to acquire information or alter it to spread harmful links or documents, luring users to interact with or download them.
- LLM jailbreaking
LLM jailbreaking involves employing intricate cues in chatbots to deceive them into responding to inquiries that violate their established rules
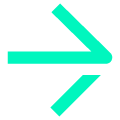
A closer look at cybercriminals’ methods
How do they do?
Injection
Creating unique input data to get around intrusion detection systems and access internal systems.
Intrusion
Directly inserting false samples into the training set to alter the behaviour of a system, such as a malware detection system.
Crowdturfing
Creating several user identities with fictitious data, the ML classifier systems are misled.
Malicious scenario example
Data transmitted to a third party make them especially vulnerable.
Prompt injection techniques can lead to unintended actions by chatbots, such as generating offensive content or disclosing confidential information.
Exhibit scammer behaviour to collect banking information.
Attackers rushing into the fog?
Threat actors don’t always have a full understanding of the model they want to target. There is a difference between black-box and white-box attacks. In black-box attack threat actors don’t know the model while in white-box attacks, attackers are well aware of the model and its parameters.